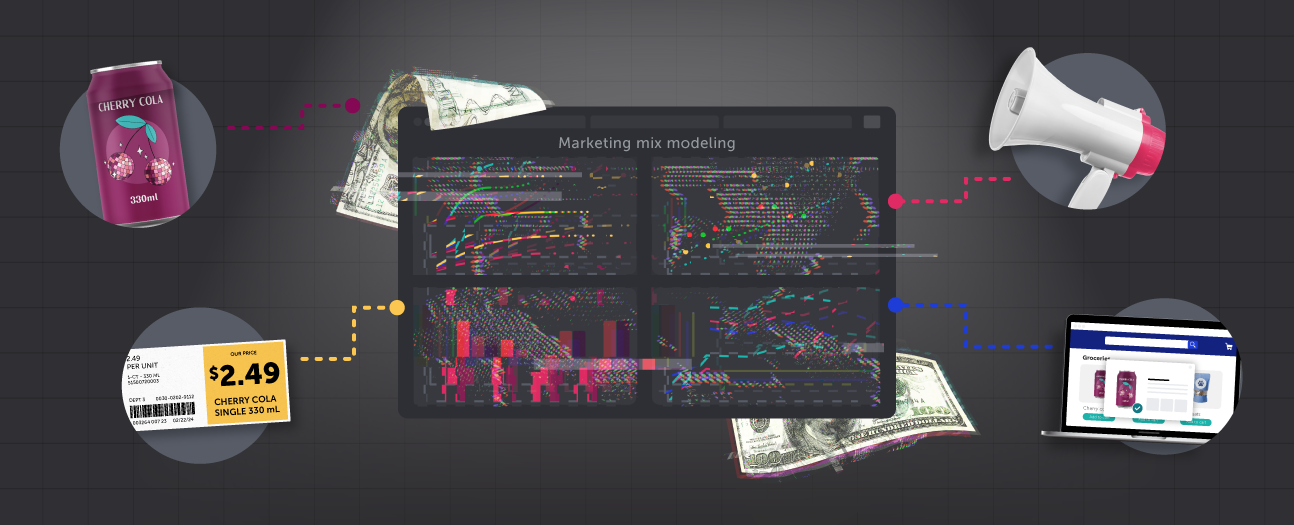
February 28, 2024
The Marketers’ Dilemma
“Only 54% of marketers are confident in ROI measurement across digital channels.”¹
This stat shouldn't be surprising — the marketing measurement landscape is rife with unreliability and indeterminability. And with increasing pressure on marketers to justify spending with data-driven business cases, several developments are making it more difficult for marketers to capture accurate attribution data: changing privacy regulations, identity resolution gaps, walled gardens, and a potentially cookie-less digital future.
Key takeaways
Is Marketing Mix Modeling the solution?
Almost half of senior marketing leaders have turned to Marketing Mix Modeling (MMM).² Before advertising’s digital evolution, MMM was the primary form of media measurement and for good reason. Its purpose was to optimize channel mix — at a time when media was bought and executed at a market or geographical level, and when deterministic measurement was just a pipe dream for most. Recall that even in the early 1990s, all hit series and major telecasts were on one of three big networks, which gave marketers access to broad reach of those markets.
Since MMM did not rely on granular tracking or targeting ability, and since 1:1 attribution with in-store purchases was deemed impossible, MMM was useful for measuring traditional upper-funnel marketing such as linear TV, radio, sponsorships, and print.
The evolution of digital marketing has expanded consumer touchpoints by an order of magnitude, enabling businesses to engage with their customers across multiple devices and platforms. Each of these touchpoints have different reach projections, no longer hitting an entire market, and in some cases, specifically designed to reach only a valuable audience segment. Linear TV, radio, and the like now work alongside programmatic display, influencer marketing, digital coupons, and so much more.
What if your data isn’t telling the right story?
In spite of the advent of digital tactics, targeting capabilities, and the ability to speak to individuals — not markets — Marketing Mix relies on data collection across a multitude of sources that creates a picture at the market level.
MMM’s main goal is to find the incremental return of every marketing investment, but critical problems arise.
- Ensuring consistent, complete, timely, quality data inputs.
- Addressing missing values in a dataset.
- Navigating different data capabilities across vendors.
Every channel has its own currencies and collection methods, making it difficult to gather data from every single channel with consistent and quality signal. Ultimately, it’s easy and quite common to run into analysis paralysis — so much data that more time is spent collecting data than producing meaningful insights in a timely fashion.
What can you do about external factors?
Marketing Mix uses statistical methods, typically multi-linear regression models instead of deterministic data — to understand how many factors combined instead of single variables in isolation affect outcomes. These regression algorithms determine the relative association of each marketing variable to sales. The corresponding coefficients are then used to calculate how many sales can be attributed to specific marketing tactics in a given time frame. The result is correlation, not causation, and again comes with challenges.
Moreover, MMM relies on multiple years of history, and models are only as reliable as the data available at the time. “New” doesn’t measure well as it can take re-development of models as data builds and becomes available, which can be expensive depending on the provider. The ever-changing data signals unlock new insights that may change decisions and chart a different course. Various digital tactics and most recently a global pandemic are examples of the curveballs that have thrown accuracy problems into nearly all MMMs. Quantifying each external factor is simply not guaranteed so assumptions are made, ones which may not hold true in practice.
Simply put, model development begins with some prior knowledge or beliefs about how certain marketing tactics affect sales. Those assumptions may include priors to constrain, calibrate or provide a baseline for the effect channels have on sales. By channel, an assumption may be made on lag between exposure and purchase or the point of diminishing returns and even long term impact.
Depending on those assumptions, the same inputs can yield different model results, and with that, different models can fit the data equally well but provide different conclusions.
What is the key to validating model accuracy?
Because cross validation isn’t possible and sole metrics like R-squared or mean absolute percentage error can be misleading, reliability becomes a concern. Furthermore, through the improper use of dummy variables these metrics can be susceptible to manipulation.
One method may include holding out a time period at the end of the data and employing the model to forecast — though can it predict what is already known? If a Bayseian frame is utilized, holdout tests can be included in the priors of the model to calibrate or in model development.
Recall that MMMs are making a substantial number of assumptions, many more than an experimental design. When a true experimental design result conflicts with a MMM, it’s typically due to flawed assumptions or data in the model. Inferential results can be supplemented with incrementality experimentation to produce credible results.
Uncover: 4 principles Ibotta uses to solve
MMM provides a very high level or top down approach to marketing channel performance — meaning you can easily lose sight of the consumer. What are you learning about the consumer’s relationship with your brand? Consumer level measurement is needed to determine which campaigns and strategies shifted specific brand KPIs.
This is why Ibotta adopted four fundamental principles to provide reliable, unbiased, actionable insights:
- Measure at the level you execute — at the user level — enabling brands and retailers to go beyond typical ROAS or iROAS metrics to provide insights into impacts to consumer behavior.
- Let the strategy determine the KPI and measure what means most to your brand based on the strategy.
- Avoid bias in methodology with a foundation in experimental design.
- Utilize the most complete, deterministic and quality data with the largest panel of verified buyers with omnichannel purchase information.
Learn more about measuring with accuracy to deeply understand your consumers and their relationship with your brand — to drive your brand forward. Contact your Ibotta rep or inquire here.
Achieve your brand goals
Which metrics are most important for your brand? Get in touch to explore possibilities with the Ibotta Performance Network.
¹Nielsen, 2023.
²Deloitte, 2022.